Passive wide-area ISR sensors can make a huge difference for warfighters
[Sponsored] A persistent stare that brings together the best combination of traditional sensors and deep learning for mission versatility.
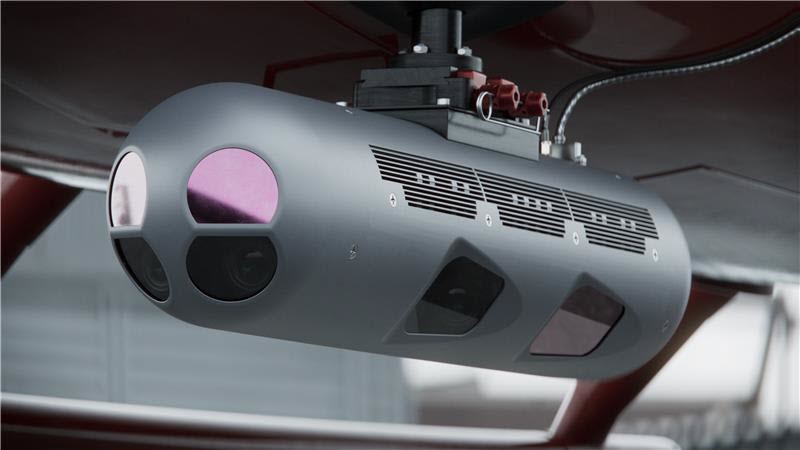
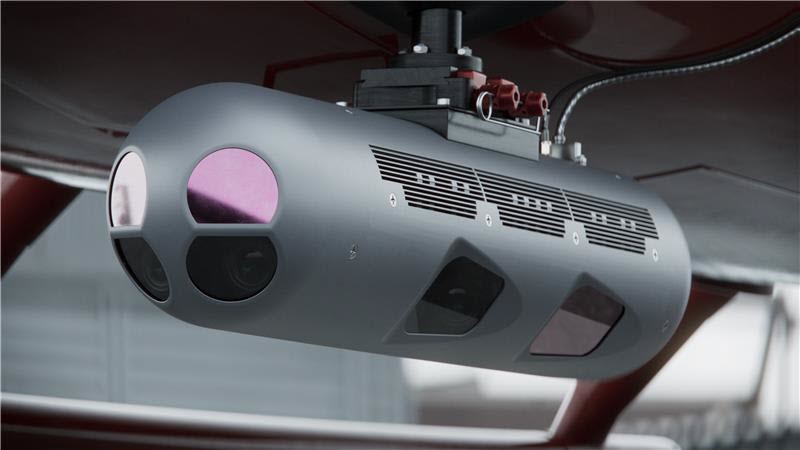
Shield AI’s ViDAR pod is completely passive, not only in the fact that it doesn’t use sensors that require emitting, it also can run completely dark to capture information without a continual communications link. (Shield AI photo)
To address the problem of active ISR sensors being easily detected in modern warfare, which can lead to the loss of costly aerial platforms, Shield AI is expanding access to ViDAR, a passive, wide-area multi-domain ISR solution originally developed by Sentient Vision Systems. In spring 2024, Shield AI acquired the Australia-based company, whose ViDAR technology is globally recognized for its cutting-edge capability, operational maturity, and proven success across maritime missions.
As a passive system, ViDAR – an acronym for visual detection and ranging – captures and stores data without emitting any signals that could be detected by adversaries. ViDAR uses a combination of traditional computer vision techniques and deep learning AI to detect, geolocate, track and classify moving and stationary targets of interest, even in challenging conditions with limited visibility.
ViDAR is currently deployed with the U.S. Marine Corps, Fisheries and Oceans Canada, Danish Home Guard, and Australian Maritime Safety Authority, demonstrating versatility across ISR, illegal fishing and search and rescue operations.
Now, Shield AI is launching the ViDAR Pod, a compact, multi-domain payload that brings this proven technology to a broader range of platforms. The pod features a 60-centimeter tube form factor, initially available as a single underbelly pod with multi-spectral cameras, an integrated processor, a separate inertial measurement unit, and industry-standard mounting options. Its unique design and advanced AI analytics allow for multi-domain (land, littoral and maritime) use within a single pod, delivering day and night coverage and the flexibility to support a wide range of mission sets.
Breaking Defense discussed the ViDAR pod with Mark Palmer, Vice President for Vision Systems at Shield AI.
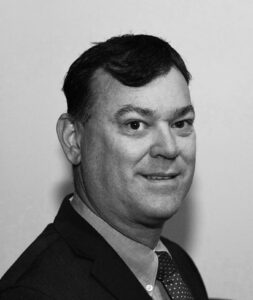
Mark Palmer is vice president for Vision Systems at Shield AI.
Breaking Defense: What is the threat scenario that the ViDAR pod addresses, and how does its passive sensing help solve warfighter problems?
Mark Palmer: What’s changed a lot for the warfighter today is the amount of active sensors that can be easily detected, and not just active sensors but just active anything – whether that be a continual communications link or traditional active sensors like radar that admit a fairly powerful signal.
We’ve become so good at detecting those elements today that we’re probably better at detecting those signals than we are at detecting or seeing the actual devices that are out there. That creates an awful problem for warfighters because as soon as they go active in any fashion, they light up a huge beacon to everybody. The end results of that can be deadly or can be loss of million-dollar drones shot out of the sky at an alarming rate by much cheaper elements. It is a large problem.
The ViDAR pod is completely passive, not only in the fact that it doesn’t use sensors that require emitting, it also can run completely dark. It can fly over an area and capture all of the information without a continual communications link. It’ll capture, process, store and then it can downlink the data either at an appropriate time, at a burst so that it’s only detectable for a very short period of time, or when it lands. This allows warfighters to be covert or to simply get the job done in an active battle zone.
I noticed that the ViDAR pod houses multiple EO/IR sensors. Tell us about its configuration.
Our concept allows for constant stare with three to five cameras that constantly cover an area rather than using a single camera that moves. The reason we do this is because that gives you absolute persistent surveillance. If you’re going through patchy clouds or through high sea levels where objects are appearing and disappearing, we will always capture them because of that constant stare.
The EO camera is a cost-effective device to put in the pod, and having several EO cameras is not a big cost increase. It’s actually cheaper to run five of these cameras than it is to run a mechanical gimbal type system. We have found that constant stare arrays provide a real benefit to the end result. LWIR cameras provide coverage for low light conditions and night missions, extending mission endurance.
Traditionally the camera array used for maritime wide area coverage versus camera configuration that are used for land detection and classification have been very different. The ViDAR pod has amalgamated these requirements together in one payload to provide real time situational awareness no matter what environment it is in. These capabilities that would typically require multiple payloads or expensive radar are packaged in a light, compact, low power solution that is suitable for Group 3 UAS, helicopters and small fixed wing aircraft, extending affordable mass to many platforms.
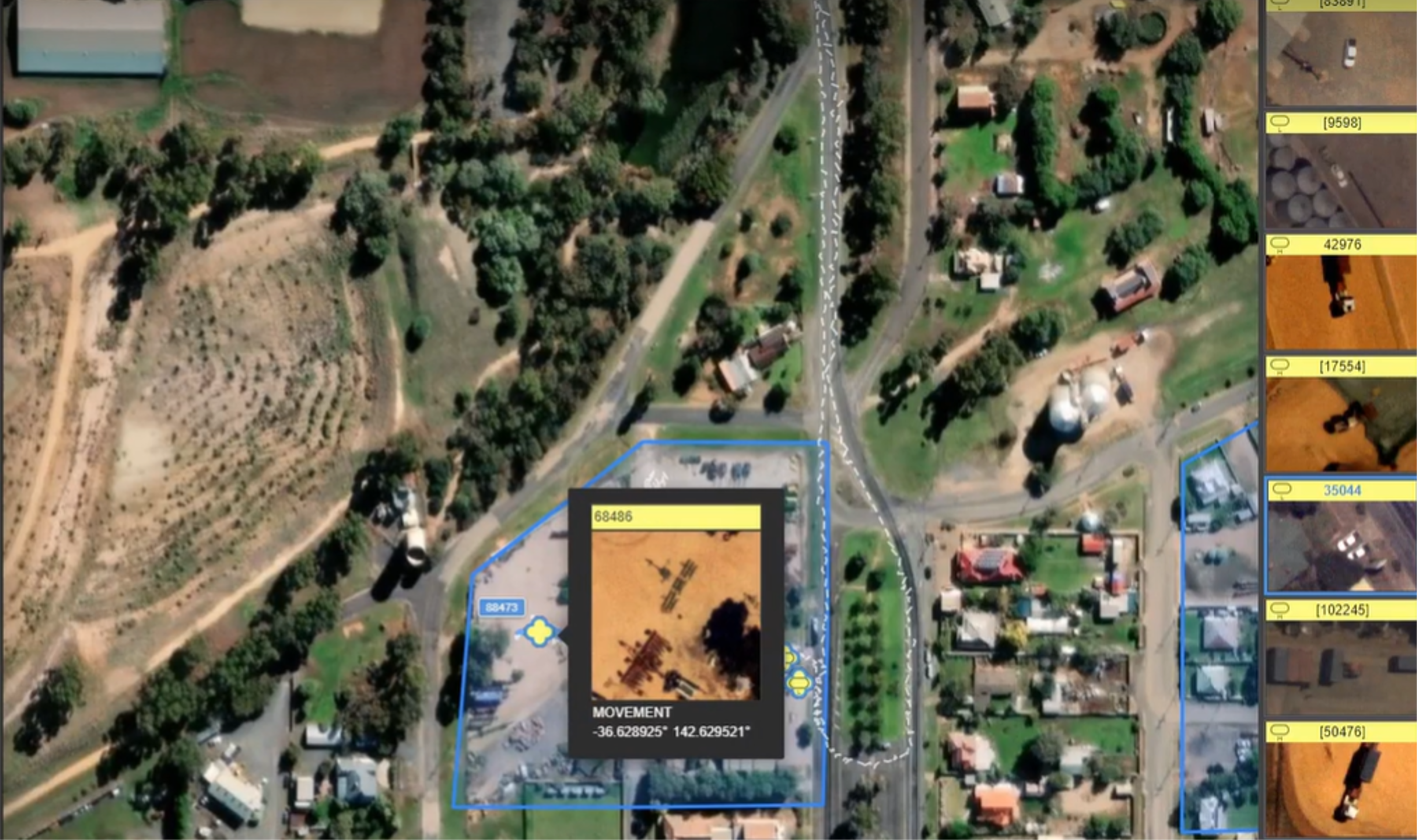
The ViDAR pod allows for constant stare with three to five cameras that constantly cover an area rather than using a single camera that moves. (Shield AI photo)
You say the artificial intelligence behind the ViDAR pod works differently than other systems on the market. How so?
The best way to answer this is to go back a bit in history. Nearly 20 years ago, Sentient Vision Systems was founded at a time when computer vision represented a very different kind of AI. Back then, it was primarily analytical – focused on detecting changes between images, identifying anomalies, and using that information to generate targets. This foundational approach laid the groundwork for modern advancements in perception-based autonomy.
We’ve moved into a new era now of deep learning where we teach the systems to recognize certain types of objects like a tank or a ship so they are able to pick those out of images. The key point with our AI is that we integrate both types of these AIs together. This is unique because we have learned through history that it’s not a good idea to just throw out some of the older capabilities.
They have particular use cases whereby they are better than some of the modern deep learning and can solve a lot of the problems that deep learning has trouble with, such as if the tank is slightly camouflaged or looks a little bit different. An AI-trained only analytic just to see something that looks like a tank will miss it. Whereas our system uses some of the traditional techniques that will still detect it. It may not classify it as a tank, but it will autonomously detect, alert with thumbnails and handover to the operator to decide, keeping the human in the loop.
Also, during poor weather or times where we have high sea states and things appear for very short periods of time, we will still be able to detect objects that are small, hard to spot, with low radar cross section because we have trained for these scenarios.
Probably the biggest advantage we have is that many modern AI systems require a lot of pixels to be able to make a successful detection. You need probably 10, 15 pixels of an object to be able to decide that is a tank.
Whereas with some of the traditional computer vision capabilities, one to two pixels is all we need for detection. That means we can detect things much further out as a potential target. As we get closer or as we can zoom in on the potential target, we can detect and classify what it is. However, we initially detect it at farther distances using traditional methods giving the operator a greater range and area of coverage.
One of our biggest advantages is also having so much diversity in our training and testing data, with systems operating all over the world and in all types of weather and lighting conditions, we have built an extensive and very operationally diverse AI solution. This diversity has been built into the system over many years of operational use and gives it the ability to be effective in real life operational situations.