AI in Supply Chain Automation: Procurement to Logistics
The adoption of AI in supply chain automation is enabling companies to make more accurate decisions, reduce cycle times, and better manage complexity. From sourcing and bid evaluation to warehouse slotting and dynamic routing, AI tools support faster and more consistent outcomes by processing large volumes of operational data and identifying patterns that human decision-makers […] The post AI in Supply Chain Automation: Procurement to Logistics appeared first on Logistics Viewpoints.
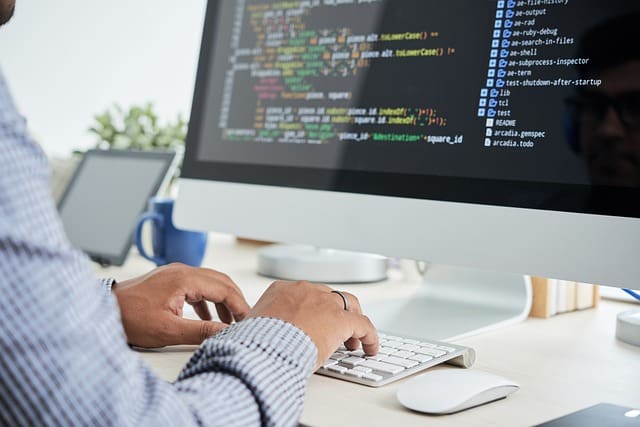
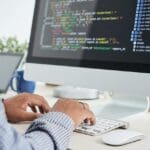
The adoption of AI in supply chain automation is enabling companies to make more accurate decisions, reduce cycle times, and better manage complexity. From sourcing and bid evaluation to warehouse slotting and dynamic routing, AI tools support faster and more consistent outcomes by processing large volumes of operational data and identifying patterns that human decision-makers may overlook. These capabilities are now being integrated into mainstream TMS, WMS, and ERP platforms.
AI in supply chain automation is gradually reshaping how core functions operate, particularly in procurement, warehousing, and logistics. Rather than acting as a full replacement for human decision-making, AI is being implemented in targeted areas where large data volumes, repeatable processes, and pattern recognition enable meaningful gains in accuracy, speed, and cost efficiency.
AI in Supply Chain Automation, Procurement, Warehouse Automation & Logistics
The integration of AI in these domains enhances decision-making and drives innovation, marking a significant shift in supply chain management, procurement, warehouse operations, and logistics. Let’s examine critical domains, review implementation considerations, and discuss realistic expectations for adoption and outcomes.
AI in Procurement: Enhancing Sourcing and Supplier Management
Procurement has traditionally relied on human expertise, manual comparison of supplier options, and analysis of past performance. The introduction of AI in supply chain automation supports procurement teams by improving access to relevant data and automating repetitive evaluation tasks.
Use Cases:
- Spend Analytics: Machine learning models analyze historical purchasing behavior to identify opportunities for cost reduction, supplier consolidation, and policy enforcement.
- Supplier Risk Monitoring: AI aggregates external signals—such as credit ratings, financial disclosures, and geopolitical events—to provide a risk profile of each vendor.
- Automated Quoting and Comparison: Natural language processing (NLP) tools extract key terms from supplier proposals and match them against RFP criteria to assist in evaluation.
- Demand Forecasting: Algorithms improve procurement planning by integrating live inputs like point-of-sale data, promotions, inventory levels, seasonality, and even weather data.
Outcomes:
- Faster sourcing cycle times and shorter RFQ-to-order workflows
- More consistent application of vendor selection criteria
- Improved risk mitigation through continuous supplier monitoring
These AI-driven tools are most effective when embedded into ERP systems and e-sourcing platforms. Integration allows seamless transitions from data insights to purchase approvals and execution.
AI in Warehouse Automation: Improving Accuracy, Throughput, and Utilization
Warehousing operations have benefited from decades of incremental automation. What distinguishes AI in warehouse automation is the ability to make real-time decisions that adapt to changing order volumes, inventory profiles, and worker conditions.
Use Cases:
- Inventory Accuracy and Slotting Optimization: AI dynamically assigns storage locations based on access frequency, item size, and order velocity to reduce picking time and improve space utilization.
- Order Picking and Packing Assistance: Vision-based AI can support robotic arms or guide workers with visual indicators and optimized pick paths that adapt to workload and floor layout.
- Predictive Maintenance for Equipment: AI tracks usage data and identifies signs of wear or potential failure in conveyor belts, forklifts, and other machinery to schedule repairs before breakdowns occur.
- Workforce Scheduling: Algorithms forecast labor needs based on inbound/outbound volume projections, product mix, and expected fulfillment deadlines.
Outcomes:
- Higher order accuracy and improved on-time performance
- Lower labor cost per unit moved and less reliance on overtime
- Reduced downtime due to more proactive equipment servicing
Many of these tools depend on inputs from sensors such as RFID tags, barcode scans, and environmental monitors. To ensure effective performance, integration with warehouse management systems (WMS) is essential.
AI in Logistics: Optimizing Routing, Freight, and Carrier Coordination
Among the most data-rich areas of the supply chain, logistics operations stand to gain significantly from AI in supply chain automation. AI systems help logistics teams manage fleet routing, freight planning, and vendor performance with greater precision.
Use Cases:
- Dynamic Route Optimization: AI models combine real-time data from GPS, traffic services, and delivery schedules to determine the most efficient routes. These systems can adjust plans mid-route in response to delays or congestion.
- Freight Cost Prediction: AI uses market history, fuel prices, and regional shipping patterns to forecast changes in freight rates and support contract negotiation strategies.
- Carrier Performance Monitoring: Past delivery data is used to generate carrier scorecards, helping logistics teams select the most reliable providers for specific lanes or timeframes.
- Exception Management: AI tools flag delayed, misrouted, or damaged shipments and recommend responses such as automatic rescheduling or inventory reallocations.
Outcomes:
- Improved delivery reliability and customer service levels
- Reduced transportation spend through route efficiency and rate optimization
- More informed selection and oversight of logistics partners
These functions are often delivered through AI modules that integrate with existing transportation management systems (TMS) or through standalone logistics platforms that connect to carrier APIs and EDI feeds.
Implementation Considerations
Despite the potential benefits, implementing AI in supply chain automation requires planning and investment in the right data infrastructure and governance processes.
- Data Quality and Integration: AI models need clean, timely, and structured data. Inconsistent data from ERP, WMS, and TMS systems must be resolved before automation will work reliably, and be trustworthy.
- Scalability: Organizations are advised to pilot AI applications in limited use cases—such as automated bid comparison or route forecasting—before scaling them across all operations.
- Change Management: AI tools often change decision-making processes or shift roles. Clear documentation, training, and stakeholder communication and buy-in are important for successful adoption.
- Cybersecurity and Ethics: AI systems require oversight to avoid data bias, improper use of personal information, or unintended automation outcomes. Human review is still necessary.
Long-Term Role of AI in Supply Chain Automation
The long-term role of AI in supply chain automation is beginning to expand beyond task-level optimization to support more strategic functions, such as network design, risk forecasting, and sustainability modeling.
Organizations that successfully deploy AI today are already seeing:
- Shorter and more efficient procurement cycles
- Higher warehouse productivity with fewer disruptions
- Better predictability in freight costs and fulfillment performance
As platforms continue to mature and interoperability improves, AI will become a standard capability embedded in core supply chain systems—supporting both day-to-day operations and long-term planning with increased confidence and clarity.
For more on the subject please read Amazon and the Shift to AI-Driven Supply Chain Planning, Walmart and the New Supply Chain Reality: AI, Automation, and Resilience and Navigating the Perfect Storm: AI Agents and Data Fabrics Empower Supply Chain Heroes Amidst Trade and AI Wars.
Remember to visit Arc Advisory Group more articles on Industrial AI like: The Rise of A2A: Completing the Industrial AI Protocol Stack with OPC UA and MCP
The post AI in Supply Chain Automation: Procurement to Logistics appeared first on Logistics Viewpoints.