For DOD, the future of large language models is smaller
Everyone loves big AI, but “maybe there is a smaller-parameter model that could run on a laptop.”
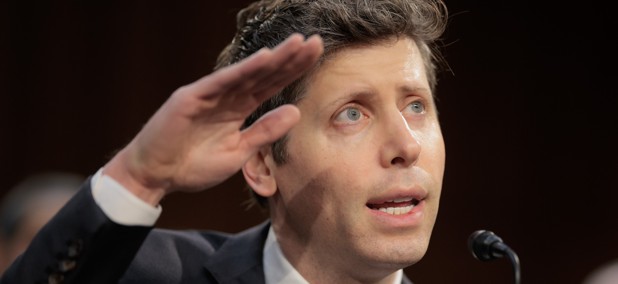
When civilians query OpenAI’s ChatGPT, for example, their answers are generated by machines trained on huge amounts of data acquired from third-party sources and millions of user interactions a day. But the government has also hired OpenAI to make need-specific tools built on smaller and more unique datasets, said Sasha Baker, OpenAI’s head of national security policy.
Baker cited the company’s recent work for U.S. national laboratories on nuclear weapons safety.
“They deal with very, very sensitive data, as you might imagine, as a nuclear lab. And so it was absolutely critical for us to figure out a way to make sure that both sides felt good about the security environment,” she said.
The company spearheaded a new form of generative AI that feels like a large language model trained on massive public datasets but that does not need to reach beyond a secure perimeter and perform tasks far more complex than writing a white paper or developing a business plan.
“We're now starting to see the first scientific papers coming out of the labs where they actually cite GPT as a contributor, or, in some cases, even a co-author, of some of the scientific work that they're doing,” Baker said.
Baker also said the company is already offering products that meet FedRAMP security requirements for secret and top-secret information.
Other AI tools are helping combatant commands to integrate vast new information streams. U.S. European Command has joined the Maven program run by the National Geospatial-Intelligence Agency with support from Palantir, said David Wilts, EUCOM’s chief data and artificial intelligence officer.
“Our previous common operating picture was fed by about three authoritative data sources. Today, over an eight- to 10-month period, we are feeding about 200 live data sources…We've stopped calling it a ‘common operational picture,’ which is a bit of an antiquated term, and we call it our decision advantage environment,” Wilts said. “We automated that data.”
This work helps to provide the intelligence that has enabled Ukraine to fight off Russian invaders, he said.
But it didn’t happen quickly or easily, he says. Training data might seem like a single, homogenous commodity but, in the case of military operational intelligence, that’s not the case.
“When I came out to EUCOM, I was excited to come out to a combatant command. I thought, ‘Boy, everything will be perfectly clear. We'll know what's going on.’ When I got here, I realized we sit on the joint data fault line, which means most of the data that we need comes from someplace else. It comes from an intelligence agency, it comes from one of the services, or it comes from an enterprise capability at the DoD level. And then we have some limited data sources here which are very, very valuable at EUCOM,” he said.
To better exchange intelligence with international partners, EUCOM set up a command-and-control integration cell to coordinate on data, AI, computer resources, people, and processes.
“If you don't have all of those systems working in unison and together, it's very hard to make progress,” said Wilts.
Especially on the computer resources, they need them in the area of responsibility and close to the area where troops will be, and where Russia is.
Once EUCOM had created that foundation—computer infrastructure close to the action, analyzing a wide variety of data—they set up what they call their “combat power laydown.” The objective is to provide a commander with a full picture of what’s going on, a picture that changes with events on the ground.
“Every given day, the commander and EUCOM need to understand what people, what assets and what munitions we have in the theater,” Wilts said. “For years, that has been a process that is almost entirely human-generated, which had previously manifested itself in a beautiful and carefully curated PowerPoint slide.”
But creating a full picture from lots of different types of data isn’t something that AI programs simply do via magic, especially when dealing with data that a specific service, office, intelligence group or even a base collected to use internally, according to specific requirements, processes, etc.
Dan Tadross, the head of public sector for Scale AI, discovered this as a Marine working with the Marine Corps Warfighting Laboratory. “All the data is in different databases. It's not clean. You don't have the context behind why that data was there. So if an aircraft was rerouted, why was it rerouted? Well, nobody was collecting that because it was in a chat system.”
It’s the sort of problem he worked on in the military, then at the Pentagon’s Joint Artificial Intelligence Center, and now at Scale AI, which has Pentagon contracts for a wide number of efforts, from advanced warplanning to assessing bomb damage to improving business workflows.
But Scale AI is also supporting Pentagon efforts that employ models or software from other AI companies. They provide AI that works to understand that chaotic mess of Defense Department data that operators, bases, offices, and convert it into something that a large software model can make sense of— in other words, to label it.
That process is critical to realizing the Pentagon’s ambition of running things like large language models or other “big” AI efforts in a way that is localized, specific, and secure from adversary attack.
In a war against an enemy with advanced electronic warfare capabilities, “I'm not going to be able to radio back to mainland or to my [air operations center] or even my advanced post,” Tadross said. “The way that Scale AI is approaching this is looking at it from the perspective of the right model for the right purpose. So maybe there is a smaller-parameter model that could run on a laptop or run on an edge device, that will still provide the added benefit of accelerating the operational planning process or accelerating the decision making process without having to rely on massive amount of like taking the data center with you to the first island chain.” ]]>