Edge Computing in Logistics: Enabling Real-Time Data Processing Closer to Operations
As logistics networks become increasingly complex, the volume of real-time data generated by devices, equipment, vehicles, and facilities is growing rapidly. Traditional cloud-centric architectures, which depend on centralized processing, may not meet speed and / or reliability goals needed to support operational needs at scale. Edge computing — processing data locally, near the source — […] The post Edge Computing in Logistics: Enabling Real-Time Data Processing Closer to Operations appeared first on Logistics Viewpoints.
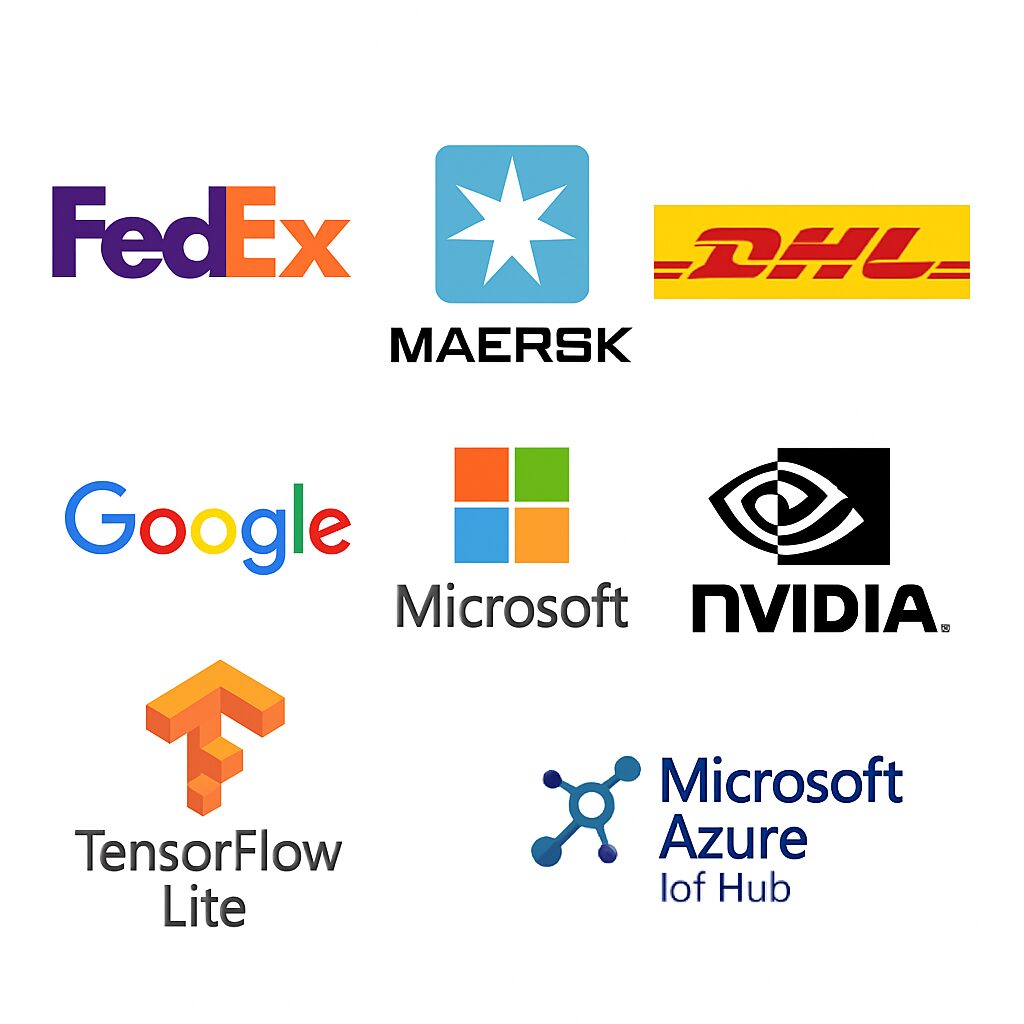
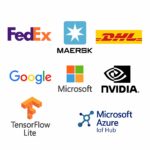
As logistics networks become increasingly complex, the volume of real-time data generated by devices, equipment, vehicles, and facilities is growing rapidly. Traditional cloud-centric architectures, which depend on centralized processing, may not meet speed and / or reliability goals needed to support operational needs at scale.
Edge computing — processing data locally, near the source — has emerged as a method to address these challenges by reducing latency and improving resiliency. However, implementing edge computing in logistics environments introduces its own set of technical and operational complexities that require careful planning.
The Challenge: Managing Complexity in Edge-Based Logistics
Deploying edge computing in logistics introduces several practical challenges. Even with local processing, network variability, particularly in remote warehouses, ports, and along mobile routes, can still cause small but impactful delays. Managing available bandwidth efficiently among many connected devices remains a continuing issue, particularly when scaling systems to significant quantities of distributed resources.
Device management is another critical area. In a typical logistics network, operators must provision, update, and monitor large fleets of devices — sensors, cameras, connected and autonomous vehicles, and mobile computers. This creates significant maintenance and oversight requirements, as each device represents a potential point of failure or vulnerability.
Data security at the edge also presents risks. Unlike centralized cloud infrastructure, edge devices are often physically accessible, making them more vulnerable to tampering or unauthorized access. Securing sensitive information — such as shipment details, customer data, and operational parameters — is a continuous concern.
A lack of industry-wide standards complicates the situation. Different manufacturers and vendors often use different protocols and systems, making integrations resource intensive from both a capital and personnel perspective. This fragmentation of connectivity often delays edge deployment initiatives.
Optimizing AI models for edge hardware is another area of difficulty. AI models designed for centralized cloud environments are often too large or power-hungry to run efficiently on smaller edge devices. Logistics organizations must carefully balance model size, speed, power consumption, and decision accuracy.
Cost also factors heavily into adoption decisions. Establishing a robust edge environment requires upfront capital investment, and demonstrating a clear return on investment is often challenging, especially in preliminary stages.
Environmental factors present additional challenges. Edge devices in logistics must often operate outdoors or in other harsh conditions, including extreme temperatures, exposure to vibration during transport, and inconsistent power quality, all of which can affect reliability.
Finally, real-time decision-making complexity must be addressed. It is not sufficient to simply collect and transmit data; edge systems must interpret and act upon data autonomously, thus introducing further technical requirements. Even after local processing, logistics organizations must ensure consistent and reliable data synchronization back to cloud-based systems for reporting and analysis without network congestion, version conflicts or other anomalies.
Addressing the Challenges: Practical Approaches
Organizations that have successfully adopted edge computing in logistics have been using a hybrid strategy, where real-time operational decisions are managed at the edge, while longer-term analytics and broader visibility are maintained in the cloud. This reduces dependency on external networks while retaining the benefits of centralized reporting and analysis.
To support this, many logistics companies are adopting AI model optimization tools designed for edge deployments, such as Google’s TensorFlow Lite and NVIDIA Jetson platforms. These allow the development of lightweight models that maintain performance within the processing and power constraints of mobile or ruggedized edge hardware.
Security strategies have also evolved. Increasingly, organizations are deploying zero-trust architectures, where each device and communication session is authenticated and encrypted by default, significantly reducing the attack surface across the network.
Managing edge devices at scale requires unified platforms capable of remote monitoring, updating, and configuration management. Solutions such as Microsoft Azure IoT Hub provide centralized oversight while maintaining device autonomy at the operational level.
Efforts to standardize data formats and interfaces are also beginning to help. Organizations like the Open Logistics Foundation and the Industrial Internet Consortium are promoting standards that reduce integration complexity across vendor ecosystems.
On the hardware side, companies are investing in ruggedized edge devices that are specifically designed to handle harsh operational environments, minimizing downtime and maintenance needs.
Operationally, many organizations are rolling out edge computing solutions in stages — starting in controlled warehouse environments where conditions are more predictable, and then expanding to more complex, mobile scenarios such as trucking or intermodal freight.
Examples of Real-World Applications
Several logistics companies have already integrated edge computing into their operations in specific use cases.
FedEx has deployed a system called SenseAwareID, which uses lightweight Bluetooth Low Energy (BLE) sensors to enable real-time package tracking without relying solely on cellular networks. Sensors communicate with edge gateways to provide operational visibility without adding significant network load.
Maersk has introduced Remote Container Management for its shipping containers. IoT sensors embedded within containers monitor environmental conditions and location data locally, transmitting only essential updates to centralized systems.
DHL has deployed smart glasses in warehouse operations as part of its Vision Picking project. The glasses process inventory and product picking data locally, reducing the time needed to retrieve and fulfill orders compared to fully cloud-dependent systems.
Key Considerations for Logistics Organizations
Several key factors should be considered when planning edge deployments in logistics.
First, edge AI differs from cloud AI in a few ways. Models must be optimized not just for accuracy, but also for constraints such as memory usage, processing speed, and battery consumption. Security must be built into the system from the outset, with a combination of hardware security modules, encryption, and continuous monitoring.
While 5G networks promise improved latency and throughput, edge computing remains important even with advanced wireless connectivity, because local processing still offers superior performance in many operational scenarios.
Progress toward interoperability is ongoing, and standards being promoted by industry groups are likely to reduce integration complexity over time.
Finally, the return on investment for edge computing often comes not from direct technology savings but from operational improvements such as reduced fulfillment cycle times, lower incident rates, more accurate inventory tracking, and improved customer service levels.
Summing Up
Edge computing is becoming a valuable tool for addressing the real-time data processing requirements of modern logistics operations. Its adoption, however, requires careful attention to technical, operational, and economic factors.
Current challenges include managing latency, device fleets, security, integration standards, AI model deployment, cost, environmental conditions, decision-making reliability, and cloud synchronization. Companies that approach these issues systematically — through hybrid architectures, AI optimization, zero-trust security, ruggedized hardware, and staged deployments — are seeing real-world operational benefits.
Use cases from FedEx, Maersk, and DHL illustrate that while edge computing deployments in logistics are still evolving, they are delivering measurable improvements in speed, reliability, and visibility.
As these technologies mature and standards emerge, edge computing is expected to play an increasingly significant role in the logistics and supply chain ecosystem both in the far and near future.
The post Edge Computing in Logistics: Enabling Real-Time Data Processing Closer to Operations appeared first on Logistics Viewpoints.